1. Introduction
An Information Retrieval System (IRS) is a vital component in the digital age, where the volume of information available is vast and ever-growing. IRSs help users find relevant information from a large repository, be it a database, library, or the internet.2. Definition and Importance of Information Retrieval
Salton, G. (1968) "An information retrieval system is a system that is capable of storage, retrieval, and maintenance of information. The system accepts requests for information from users and retrieves the required information." Van Rijsbergen, C. J. (1979) "Information retrieval is concerned with the organization and retrieval of information from a large number of text-based documents. It is essentially a problem of selection, and the main concern is to devise appropriate representations of the documents and the information need to facilitate the selection process." Croft, W. B., Metzler, D., & Strohman, T. (2010) "Information retrieval systems are designed to help find information stored in computers. It is the process of obtaining information system resources that are relevant to an information need from a collection of those resources." Information Retrieval (IR) refers to obtaining information from an extensive repository of resources relevant to an information need, usually expressed as a query by the user. The importance of IR lies in its ability to:-
• Enhance Access: Facilitate access to vast amounts of data.
• Improve Decision-Making: Provide relevant information for informed decision-making.
• Boost Efficiency: Save time by retrieving pertinent information quickly.
3. Components of an Information Retrieval System
An IRS consists of several vital components that retrieve relevant information based on user queries. These components include:-
a) Document Collection:
-
• The repository or database containing the information to be retrieved. It can include various formats such as text documents, multimedia files, web pages, etc.
• Examples: Digital libraries, corporate databases, and web archives.
-
• Types of Indexing:
-
o Inverted Index: Maps terms to their locations in the document collection.
o Forward Index: Maps documents to the terms they contain.
-
o Tokenisation: Breaking text into individual terms or tokens.
o Stemming: Reducing words to their root forms (e.g., "running" to "run").
o Stop Word Removal: Removing common but unimportant words (e.g., "the," "is").
-
• Query Parsing: Breaking down the query into individual components.
• Query Expansion: Enhancing the query with additional terms or synonyms to improve retrieval.
• Relevance Feedback: Modifying the query based on user feedback to retrieve more relevant results.
-
• Boolean Model: Uses logical operators (AND, OR, NOT) to match documents to the query.
• Vector Space Model: Represents documents and queries as vectors in a multi-dimensional space and measures similarity.
• Probabilistic Model: Estimates the probability that a document is relevant to the query.
-
• Term Frequency-Inverse Document Frequency (TF-IDF): Measures the importance of a term in a document relative to its frequency across the collection.
• PageRank: Google uses it to rank web pages based on their link structure.
• BM25: A probabilistic relevance ranking function.
-
• Search Box: Allows users to enter their queries.
• Results Display: A list of retrieved documents, often with snippets and highlights.
• Advanced Search Options: Provides filters and additional parameters to refine the search.
4. Types of Information Retrieval Systems
IRSs can be categorised based on their application, scope, and technology. Some common types include:-
a) Web Search Engines: Systems designed to retrieve information from the internet. Examples: Google, Bing, DuckDuckGo. Main features: Crawling, indexing web pages, and handling large-scale queries.
b) Enterprise Search Systems: Designed to retrieve information within an organisation. Main features: security controls, integration with corporate databases, and support for various file formats. Examples: Elasticsearch, Apache Solr.
c) Digital Libraries: Specialized systems for managing and retrieving academic and research information. Features: Metadata indexing, support for scholarly articles, and citation tracking. Examples: PubMed, IEEE Xplore.
d) Multimedia Retrieval Systems: Systems that handle multimedia content like images, videos, and audio. Features: Content-based retrieval, metadata tagging, and machine learning for pattern recognition. Examples: YouTube search, Google Images.
5. Working Principles of Information Retrieval Systems
An IRS operates based on several principles and algorithms designed to optimise the retrieval process. Key principles include:-
a) Relevance: The measure of how well a document meets the user's information needs. Factors Influencing Relevance:
-
• Content Matching: How closely the document content matches the query terms.
• Context: The context in which the terms are used within the document.
• User Preferences: Historical data on user behaviour and preferences.
-
• Precision: The ratio of relevant documents retrieved to the total documents retrieved.
• Recall: The ratio of relevant documents retrieved to the total relevant documents in the collection.
• Trade-off: Often, increasing precision reduces recall and vice versa. Balancing both is crucial for effective retrieval.
-
• BM25: A probabilistic retrieval model that scores documents based on term and inverse document frequency.
• TF-IDF: Scores documents based on the frequency of query terms in the document and their rarity in the collection.
• PageRank: Scores web pages based on their link structure, giving higher importance to pages with more incoming links.
-
• Adding related terms or synonyms to the original query to cover a broader search scope.
• Relevance Feedback: Using user feedback on the relevance of retrieved documents to refine the query and improve results.
-
• Named Entity Recognition (NER): Identifying and classifying proper names in text.
• Part-of-Speech Tagging: Labeling words with their grammatical roles.
• Sentiment Analysis: Determining the sentiment expressed in the text.
6. Evaluation of Information Retrieval Systems
Evaluating the effectiveness of an IRS is critical to ensure it meets user needs and performs optimally. Standard evaluation metrics and methods include:-
a) Precision and Recall:
-
• Precision: Measures the accuracy of the retrieved documents.
• Recall: Measures the completeness of the retrieval process.
• F-Measure: A harmonic mean of precision and recall, balancing both metrics.
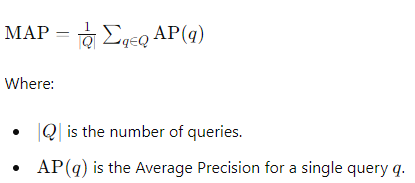
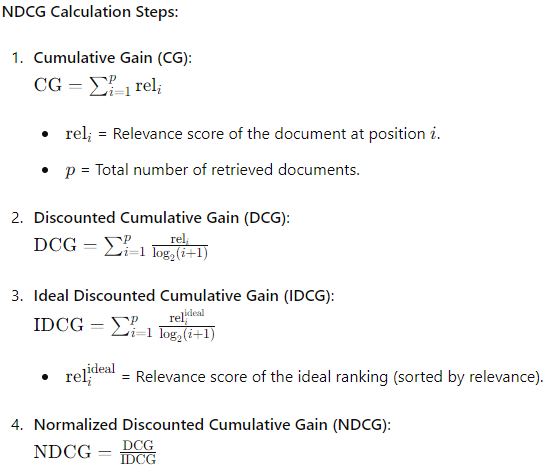
-
• Surveys: Collecting user feedback through questionnaires.
• Usage Analytics: Analyzing user interactions and behavior within the system.
-
• Response Time: Time taken to retrieve results.
• Throughput: Number of queries processed in a given time frame.
• Scalability: Ability to handle growing amounts of data and users.
7. Current Trends in Information Retrieval
The field of IR is continually evolving, with several emerging trends shaping the future of IRSs. Some of the notable trends include:-
a) Machine Learning and AI: Integrating machine learning and artificial intelligence (AI) techniques to enhance IR. Applications:
-
• Rank Learning: Training models to learn optimal ranking functions.
• Personalization: Customizing search results based on user preferences and behaviour.
• Content Analysis: Using AI to understand and categorise content, such as image recognition and sentiment analysis.
-
• Entity Recognition: Identifying and linking entities within queries and documents.
• Knowledge Graphs: Using structured data to provide context and relationships between entities.
• Natural Language Understanding (NLU): Interpreting the intent and nuances of user queries.
-
• Scalability: Ability to process and store massive amounts of data.
• Performance: Enhanced computational power and storage capabilities.
• Flexibility: Access to a wide range of tools and services for data processing and analysis.
-
• Voice Recognition: Understanding and processing spoken queries.
• Natural Language Processing: Engaging in dialogues to refine queries and provide more accurate results.
• Context Awareness: Remembering previous interactions to improve the search experience.
-
• Encryption: Protecting data during storage and transmission.
• Access Controls: Implementing authentication and authorisation mechanisms.
• Privacy-Preserving IR: Techniques to perform IR without compromising user privacy.
8. Information Retrieval System and Knowledge Management
Information Retrieval Systems (IRS) and Knowledge Management (KM) are interrelated domains that collectively enhance the capability of organisations to manage, access, and utilise information. This document provides an in-depth exploration of the IRS, its integration into KM, and the effects of this integration on organisational efficiency, decision-making, and innovation.9. Understanding Information Retrieval Systems (IRS)
An Information Retrieval System (IRS) is designed to collect, organise, and facilitate information retrieval. Its primary purpose is to help users find relevant information from a vast repository based on specific queries. Critical components of an IRS include:-
a) Document Collection: The database or repository containing the information.
b) Indexing: Creating a structured representation of the document collection to enable efficient search and retrieval.
c) Query Processing: The mechanism by which user queries are interpreted and processed.
d) Search and Retrieval: The core function of finding and retrieving documents that match the query.
e) Ranking and Relevance: Ordering the retrieved documents based on their relevance to the query.
f) User Interface: The platform through which users interact with the system.
10. Knowledge Management (KM)
Knowledge Management (KM) involves the systematic process of creating, sharing, using, and managing the knowledge and information of an organisation. KM aims to enhance organisational learning, innovation, and performance by ensuring that valuable knowledge is available to those who need it. Critical activities in KM include:-
a) Knowledge Creation: Developing new knowledge through research, innovation, and collaboration.
b) Knowledge Storage and Retrieval: Storing knowledge in repositories and making it retrievable.
c) Knowledge Sharing: Disseminating knowledge across the organisation.
d) Knowledge Application: Using knowledge to improve processes, products, and services.
11. Integration of IRS in Knowledge Management
Integrating IRS into KM systems enhances organisations' ability to effectively manage and utilise their knowledge assets. This integration impacts various aspects of KM, including knowledge creation, storage, sharing, and application.-
a) Enhanced Knowledge Access and Retrieval: IRS improves the accessibility and retrieval of knowledge by:
-
• Efficient Search Capabilities: Enabling users to find relevant information from large repositories quickly.
• Advanced Query Processing: Allowing complex queries that can pinpoint specific information needs.
• Improved Indexing: Ensuring that knowledge is well-organized and easily searchable.
-
• Reduced Search Time: Employees spend less time searching for information, leading to increased productivity.
• Better Decision-Making: Quick access to relevant information supports informed decision-making.
• Increased Knowledge Utilization: Easier access to knowledge encourages its use across the organisation.
-
• Metadata Utilization: IRS employs metadata to describe, classify, and index knowledge resources.
• Structured Data Management: Ensures that knowledge is stored structured, facilitating efficient retrieval and use.
-
• Enhanced Knowledge Discovery: Well-organized knowledge repositories make discovering new and relevant information more accessible.
• Streamlined Knowledge Management Processes: Efficient organisation reduces the complexity of managing large volumes of knowledge.
-
• Comprehensive Information Access: Facilitates access to diverse information sources, fostering creativity and innovation.
• Interdisciplinary Research: Enables cross-disciplinary information retrieval, supporting innovative research and development.
-
• Accelerated Innovation: Access to comprehensive information resources speeds up innovation.
• Enhanced Collaboration: Facilitates collaborative efforts by providing a shared platform for information access.
-
• Centralized Knowledge Repositories: Provide a single access point for all knowledge resources.
• Collaborative Tools: Integration with collaborative tools like wikis, forums, and social platforms enhances knowledge sharing.
-
• Improved Knowledge Dissemination: Centralized repositories and collaborative tools make sharing knowledge across the organisation easier.
• Stronger Knowledge Networks: Facilitates the development of robust knowledge networks and communities of practice.
-
• Contextual Information Retrieval: Provides context-aware search results relevant to the user's needs.
• Decision Support Systems: Integrates with decision support systems to provide relevant knowledge for decision-making processes.
-
• Better Utilization of Knowledge: Ensures that knowledge is applied effectively to improve processes, products, and services.
• Increased Organizational Agility: Quick access to applicable knowledge enhances the organisation's ability to respond to changes and challenges.
12. Case Studies and Examples
-
a) Google Search Engine and Knowledge Management: Google's search engine is one of the most advanced IRS, providing powerful search capabilities that support KM in various ways:
-
• Comprehensive Indexing: Google's extensive indexing capabilities ensure that vast information is easily searchable.
• Advanced Query Processing: Google's algorithms can interpret complex queries and provide relevant results.
• Personalized Search: Google uses user data to personalise search results, enhancing relevance and utility.
-
• Global Knowledge Sharing: Google facilitates global access to knowledge, supporting worldwide KM efforts.
• Research and Innovation: Researchers and innovators can access information, fostering discoveries and developments.
-
• Scalability: Can handle large volumes of data, making it suitable for large organisations.
• Customizable Search: Allows organisations to tailor the search experience to their needs.
• Integration with KM Tools: Can be integrated with other KM tools and systems for seamless knowledge management.
-
• Enhanced Internal Knowledge Access: Employees can quickly find the necessary information, improving efficiency and productivity.
• Improved Collaboration: Centralized search capabilities support collaborative efforts by making shared knowledge easily accessible.
13. Future Trends and Developments
The integration of IRS and KM is an evolving field with several emerging trends and developments:-
a) Artificial Intelligence and Machine Learning: AI and machine learning transform IRS and KM by providing more intelligent and adaptive systems.
-
• Smart Search: AI-driven search engines can understand natural language queries and provide more accurate results.
• Predictive Analytics: Machine learning algorithms can predict information needs and proactively suggest relevant knowledge.
-
• Enhanced Search Accuracy: AI improves the accuracy of search results, making knowledge retrieval more efficient.
• Proactive Knowledge Management: Predictive analytics can identify potential knowledge gaps and suggest areas for improvement.
-
• Context-Aware Retrieval: Semantic search engines can interpret the meaning of queries and provide contextually relevant results.
• Knowledge Graphs: Structured representations of knowledge that show relationships between concepts and entities.
-
• Improved Knowledge Discovery: Semantic search and knowledge graphs enhance the ability to discover and understand complex information.
• Better Decision-Making: Context-aware retrieval provides more relevant information for decision-making processes.
-
• Unified Platforms: Combining search capabilities with collaboration tools like Slack, Microsoft Teams, and Confluence.
• Real-Time Collaboration: Facilitates real-time knowledge sharing and collaboration across teams and departments.
-
• Seamless Knowledge Sharing: Integration with collaboration tools ensures that knowledge can be easily shared and accessed in real time.
• Enhanced Team Collaboration: Unified platforms support more effective collaboration, leading to better outcomes and innovation.
14. Conclusion
Integrating Information Retrieval Systems into Knowledge Management is crucial for organisations aiming to maximise the utility of their knowledge assets. IRS enhances KM by improving knowledge access, organisation, creation, sharing, and application. Advanced search capabilities, efficient indexing, and contextual information retrieval support better decision-making, innovation, and collaboration. Emerging trends like AI, semantic search, and integration with collaboration tools are set to revolutionise the field further, making KM more effective and efficient.15. References
-
Baeza-Yates, R., & Ribeiro-Neto, B. (2011). Modern Information Retrieval: The Concepts and Technology behind Search (2nd ed.). Addison-Wesley.
Croft, W. B., Metzler, D., & Strohman, T. (2015). Search Engines: Information Retrieval in Practice. Pearson.
Manning, C. D., Raghavan, P., & Schütze, H. (2008). Introduction to Information Retrieval. Cambridge University Press.
Salton, G., & McGill, M. J. (1983). Introduction to Modern Information Retrieval. McGraw-Hill.
Singhal, A. (2001). Modern information retrieval: A brief overview. IEEE Data Engineering Bulletin, 24(4), 35-43.
Zobel, J., & Moffat, A. (2006). Inverted files for text search engines. ACM Computing Surveys (CSUR), 38(2), 1-56.
Salton, G. (1968). Automatic Information Organization and Retrieval. New York: McGraw-Hill.
Van Rijsbergen, C. J. (1979). Information Retrieval (2nd ed.). London: Butterworths.
Croft, W. B., Metzler, D., & Strohman, T. (2010). Search Engines: Information Retrieval in Practice. Boston: Addison-Wesley.